일 | 월 | 화 | 수 | 목 | 금 | 토 |
---|---|---|---|---|---|---|
1 | 2 | 3 | 4 | |||
5 | 6 | 7 | 8 | 9 | 10 | 11 |
12 | 13 | 14 | 15 | 16 | 17 | 18 |
19 | 20 | 21 | 22 | 23 | 24 | 25 |
26 | 27 | 28 | 29 | 30 | 31 |
- Absolute
- AGI
- ai
- AI agents
- AI engineer
- AI researcher
- ajax
- algorithm
- Algorithms
- aliases
- Array 객체
- ASI
- bayes' theorem
- Bit
- Blur
- BOM
- bootstrap
- canva
- challenges
- ChatGPT
- Today
- In Total
목록전체 글 (554)
A Joyful AI Research Journey🌳😊
ChatGPT, OpenAIFor text generation, the evaluation metric often depends on the specific task and desired outcomes. However, some common evaluation metrics used in NLP for text generation tasks include:Perplexity:Definition: Perplexity measures how well a probability model predicts a sample. In the context of language models, lower perplexity indicates a better predictive model.Usage: It is widel..
ChatGPT, OpenAIYes, using Retrieval-Augmented Generation (RAG) would indeed be a better choice for the scenario where you want to write prompts like "write a positive review about a certain movie" or "write a negative review about a certain movie." Here’s why RAG is more suitable for this task:1. Contextual Relevance and Specificity:RAG can retrieve specific reviews or information related to the..
ChatGPT, OpenAIPretraining GPT-2 with Rotten Tomatoes data and incorporating Retrieval-Augmented Generation (RAG) with the same data are two different approaches with distinct goals and outcomes. Here’s a breakdown of the differences:1. Pretraining or Fine-Tuning GPT-2 with Rotten Tomatoes DataWhat It Is:Pretraining: Training GPT-2 from scratch using a large corpus like Rotten Tomatoes data (not..
The * in zip(*combined_dataset) is the "unpacking" operator in Python. It takes a list of tuples (in this case, combined_dataset, which consists of pairs like (review_text, label)) and "unzips" them into two separate tuples: one for texts and one for labels.In other words:texts will contain all the review texts.labels will contain all the corresponding labels.The * operator effectively transpose..
Join two tuples together:a = ("John", "Charles", "Mike")b = ("Jenny", "Christy", "Monica")x = zip(a, b)#use the tuple() function to display a readable version of the result:print(tuple(x))(('John', 'Jenny'), ('Charles', 'Christy'), ('Mike', 'Monica'))https://www.w3schools.com/python/ref_func_zip.asp W3Schools.comW3Schools offers free online tutorials, references and exercises in all the major la..
The model bert-base-uncased is used because it converts all text to lowercase before processing, ignoring case differences. This is particularly useful when case sensitivity is not important for the task, such as sentiment analysis, where "Happy" and "happy" should be treated the same. The "uncased" version is generally more efficient and performs well when the distinction between uppercase and ..
ChatGPT, OpenAINaive Bayes in Sentiment Analysis:Pros:Simplicity: Easy to implement and interpret.Efficiency: Works well with smaller datasets and requires less computational power.Baseline: Provides a strong baseline for comparison with more complex models.Cons:Assumption of Independence: Assumes features (words) are independent, which is often not true in language processing.Limited Understand..
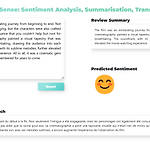
ChatGPT, OpenAIHelsinki-NLP (OPUS-MT):Pros:Lightweight: Generally smaller models, making them easier to deploy with lower computational resources.Accessibility: Open-source and widely accessible with many pre-trained models available.Specialized: Many models are specialized for specific language pairs, providing good performance for those tasks.Cons:Performance: May not perform as well on comple..
https://huggingface.co/facebook/mbart-large-50-many-to-many-mmt facebook/mbart-large-50-many-to-many-mmt · Hugging FacemBART-50 many to many multilingual machine translation This model is a fine-tuned checkpoint of mBART-large-50. mbart-large-50-many-to-many-mmt is fine-tuned for multilingual machine translation. It was introduced in Multilingual Translation with Extensiblhuggingface.cohttps://h..
https://medium.com/@sandyeep70/demystifying-text-summarization-with-deep-learning-ce08d99eda97 Text Summarization with BART ModelIntroductionmedium.comdef text_summarizer_from_pdf(pdf_path): pdf_text = extract_text_from_pdf(pdf_path) model_name = "facebook/bart-large-cnn" model = BartForConditionalGeneration.from_pretrained(model_name) tokenizer = BartTokenizer.from_pretrained(model_..