์ผ | ์ | ํ | ์ | ๋ชฉ | ๊ธ | ํ |
---|---|---|---|---|---|---|
1 | 2 | 3 | 4 | 5 | 6 | 7 |
8 | 9 | 10 | 11 | 12 | 13 | 14 |
15 | 16 | 17 | 18 | 19 | 20 | 21 |
22 | 23 | 24 | 25 | 26 | 27 | 28 |
29 | 30 | 31 |
- Absolute
- AGI
- ai
- AI agents
- AI engineer
- AI researcher
- ajax
- algorithm
- Algorithms
- aliases
- Array ๊ฐ์ฒด
- ASI
- bayes' theorem
- Bit
- Blur
- BOM
- bootstrap
- canva
- challenges
- ChatGPT
- Today
- In Total
A Joyful AI Research Journey๐ณ๐
[4] 241104 Data Preprocessing, Word Cloud, ChatGPT API [Goorm All-In-One Pass! AI Project Master - 4th Session, Day 4] ๋ณธ๋ฌธ
[4] 241104 Data Preprocessing, Word Cloud, ChatGPT API [Goorm All-In-One Pass! AI Project Master - 4th Session, Day 4]
yjyuwisely 2024. 11. 4. 11:53241104 Mon 4th class
์ค๋ ๋ฐฐ์ด ๊ฒ ์ค ๊ธฐ์ตํ ๊ฒ์ ์ ๋ฆฌํ๋ค.
https://rowan-sail-868.notion.site/da602ba0748c4e2cb12b443178b16507
ํ ์คํธ ๋ฐ์ดํฐ ๋ถ์ ๊ธฐ๋ณธ | Notion
์์ ์๋ฃ๋ก ์ ์ํ๊ธฐ
rowan-sail-868.notion.site
https://ldjwj.github.io/CHATGPT_AI_CLASS/01_TextPre_V10.html
01_TextPre_V10
ldjwj.github.io
๋น์ ๊ณต์, ๋ํ์X, 4๋
๋์ ์ฌ๋ผ๊ฐ, ๋ํ 2๊ฐ 1๋ฑ
์ง์คํด์ ๊ณต๋ถ
ํ๋๋ฅผ ํ๋๋ผ๋ ์ ๋๋ก ํ๋ค.
๋ ผ๋ฌธ ์ฝ๊ณ ๋ํ ์ฐธ์ฌ, ํผ์ ๊ณต๋ถ, ์ฑ์ฅํจ
๋ฆ์ ๊ฑด X
์๋ค๊ฐ๋ค X ์ฐ๋ฃจ๋ฃจ ์ซ์๋ค๋์ง X
๋๊ธฐ์ ๋ฌธ์ ๋ง์
๊ณผ์ 2-3๊ฐ ํ๋ค๊ณ ์ง์ ๋ง์์ง๋ ๊ฑด ์๋๋ค
์ด๊ธ ๊ณผ์ ์ ๋น์ทํ๋ค
1๋ ๊ณต๋ถํด๋ ๋ฌ๋ผ์ง ๊ฒ ์๋ค ๋์ ๊ฑด X
๋ง์ด ์์์ผ ํ๋ค
ํ์ ๋ผ์ด๋ธ๋ฌ๋ฆฌ๋ ํ์๋ค.
์ด์ฌํ ํ๋ค
01. ์์ฐ์ด ์ฒ๋ฆฌ(NLP)๋ ๋ฌด์์ธ๊ฐ?
- ์์ฐ์ด ์ฒ๋ฆฌ(NLP)๋ ์ธ๊ฐ์ ์ธ์ด๋ฅผ ์ปดํจํฐ๊ฐ ์ดํดํ๊ณ ์ฒ๋ฆฌํ ์ ์๋๋ก ํ๋ ๊ธฐ์
- NLP๋ ํ ์คํธ์ ์์ฑ ๋ฐ์ดํฐ๋ฅผ ๋ถ์ํ๊ณ , ์ด๋ฅผ ํตํด ์๋ฏธ๋ฅผ ์ถ์ถํ๊ฑฐ๋ ์๋ก์ด ํ ์คํธ๋ฅผ ์์ฑํ๋ ๋ค์ํ ๋ฐฉ๋ฒ์ ํฌํจ.
03. ์์ฐ์ด ์ฒ๋ฆฌ์ ๊ธฐ๋ณธ ์ฉ์ด๋ฅผ ์ดํด
- ํ ํฐํ(Tokenization): ํ ์คํธ๋ฅผ ์์ ๋จ์๋ก ๋ถํ .
- ํํ์ ๋ถ์(Morphological Analysis): ๋จ์ด๋ฅผ ํํ์๋ก ๋ถ๋ฆฌํ๊ณ ํ์ฌ ํ๊น .
- ์ด๊ฐ ์ถ์ถ(Stem Extraction): ๋จ์ด์ ๊ธฐ๋ณธ ํํ ์ถ์ถ.
- ํ์ฌ ํ๊น (part-of-speech tagging (POS) Tagging): ๋จ์ด์ ํ์ฌ๋ฅผ ์๋ณ.
- ๋ช ๋ช ๋ ๊ฐ์ฒด ์ธ์(NER): ์ธ๋ฌผ, ์ฅ์ ๋ฑ์ ๋ช ๋ช ๋ ๊ฐ์ฒด ์๋ณ.
- ๋จ์ด ์๋ฒ ๋ฉ(Word Embedding): ๋จ์ด๋ฅผ ๋ฒกํฐ๋ก ๋ณํํ์ฌ ์๋ฏธ๋ฅผ ์์น์ ์ผ๋ก ํํ.
ํ ์คํธ๋ฐ์ดํฐ๋ถ์1_๋น๋๋ถ์_V10
ldjwj.github.io
๋น๋ ๋ถ์
LLM ์ฑ ์ ๋ค ๋์จ๋ค.
import nltk
print(nltk.__version__)
3.8.1
!pip install nltk
Requirement already satisfied: nltk in /usr/local/lib/python3.10/dist-packages (3.8.1) Requirement already satisfied: click in /usr/local/lib/python3.10/dist-packages (from nltk) (8.1.7) Requirement already satisfied: joblib in /usr/local/lib/python3.10/dist-packages (from nltk) (1.4.2) Requirement already satisfied: regex>=2021.8.3 in /usr/local/lib/python3.10/dist-packages (from nltk) (2024.9.11) Requirement already satisfied: tqdm in /usr/local/lib/python3.10/dist-packages (from nltk) (4.66.6)
import nltk
from nltk.tokenize import word_tokenize
from nltk.tag import pos_tag
# NLTK ๋ฐ์ดํฐ ๋ค์ด๋ก๋ (์ฒ์ ํ ๋ฒ๋ง ์คํ)
nltk.download('punkt')
nltk.download('averaged_perceptron_tagger')
[nltk_data] Downloading package punkt to /root/nltk_data...
[nltk_data] Unzipping tokenizers/punkt.zip.
[nltk_data] Downloading package averaged_perceptron_tagger to
[nltk_data] /root/nltk_data...
[nltk_data] Unzipping taggers/averaged_perceptron_tagger.zip.
True
# ์์ ๋ฌธ์ฅ
sentence = "Natural language processing makes it possible for computers to understand human language."
# ํ ํฐํ (Tokenization)
tokens = word_tokenize(sentence)
print("ํ ํฐํ ๊ฒฐ๊ณผ:", tokens)
# ํ์ฌ ํ๊น
(POS Tagging)
tagged_tokens = pos_tag(tokens)
print("ํ์ฌ ํ๊น
๊ฒฐ๊ณผ:", tagged_tokens)
ํ ํฐํ ๊ฒฐ๊ณผ: ['Natural', 'language', 'processing', 'makes', 'it', 'possible', 'for', 'computers', 'to', 'understand', 'human', 'language', '.']
ํ์ฌ ํ๊น
๊ฒฐ๊ณผ: [('Natural', 'JJ'), ('language', 'NN'), ('processing', 'NN'), ('makes', 'VBZ'), ('it', 'PRP'), ('possible', 'JJ'), ('for', 'IN'), ('computers', 'NNS'), ('to', 'TO'), ('understand', 'VB'), ('human', 'JJ'), ('language', 'NN'), ('.', '.')]
re — Regular expression operations
Source code: Lib/re/ This module provides regular expression matching operations similar to those found in Perl. Both patterns and strings to be searched can be Unicode strings ( str) as well as 8-...
docs.python.org
F4
๋ถ์ฉ์ด์ฒ๋ฆฌ
๋ถ์ฉ์ด ๋๋ ์ ์ธ์ด๋ ๋ถ์ฉ ๋ชฉ๋ก์ ์๋ ๋จ์ด๋ก, ์์ฐ์ด ๋ฐ์ดํฐ ์ฒ๋ฆฌ ์ ํ์ ์ค์ํ์ง ์๊ธฐ ๋๋ฌธ์ ํํฐ๋ง๋๋ค. ๋ชจ๋ ์์ฐ์ด ์ฒ๋ฆฌ ๋๊ตฌ์์ ์ฌ์ฉ๋๋ ๋จ์ผํ ๋ฒ์ฉ ๋ถ์ฉ์ด ๋ชฉ๋ก์ ์์ผ๋ฉฐ, ๋ถ์ฉ์ด ์๋ณ์ ์ํด ํฉ์๋ ๊ท์น๋ ์์ผ๋ฉฐ, ์ค์ ๋ก ๋ชจ๋ ๋๊ตฌ๊ฐ ์ด๋ฌํ ๋ชฉ๋ก์ ์ฌ์ฉํ๋ ๊ฒ๋ ์๋๋ค.
import os #๋ฆฌ๋
์ค: ํด๋ ์ด๋ฆ directory
from collections import Counter #๋น๋ ๊ฐ์ ํ์ธ
import re
from nltk.corpus import stopwords #์์ด, ๋ถ์ฉ์ด ๋ชจ์๋
import matplotlib.pyplot as plt
# ํ
์คํธ ํ์ผ ๊ฒฝ๋ก
file_paths = [
"01_๋ค๋ฅธ๊ฒฝ์์ฌ์๊ฐ๋จ๋น๊ต.txt",
"02_๊ธฐ์
๋ฆฌ์์น๊ด๋ จ์ ๋ฆฌ.txt",
"03_์์ฑAI๋ถ์.txt"
]
for file_path in file_paths:
with open(file_path, 'r', encoding='utf-8') as file: # r ์ฝ๊ธฐ ์ ์ฉ
text = file.read()
print("ํ์ผ๋ช
: ", file_path)
print("ํ์ผ ๋ด์ฉ : ", text)
# ํ๊ตญ์ด ๋ถ์ฉ์ด ์ถ๊ฐ
# ์๋์ผ๋ก ์ ์ํ ํ๊ตญ์ด ๋ถ์ฉ์ด ๋ฆฌ์คํธ
korean_stopwords = {
'์', '๊ฐ', '์ด', '์', '๋ค', '๋', '์ข', '์', '๊ฑ', '๊ณผ', '๋', '๋ฅผ', '์ผ๋ก',
'์', '์', '์', 'ํ', 'ํ๋ค', '์์', '๊ฒ', '๋ฐ', '์ํด', '๊ทธ', '๋๋ค'
}
additional_stopwords = {'๊ฐ์ ', '์ฝ์ ', '๊ฒฝ์์ฌ'} # ๋ถ์์ ๋ถํ์ํ ๋จ์ด ์ถ๊ฐ
korean_stopwords.update(additional_stopwords)
# ํ์ผ๋ณ ํ
์คํธ ์ฒ๋ฆฌ ๋ฐ ๋จ์ด ๋น๋ ๊ณ์ฐ
def process_text(text):
# ํ
์คํธ ์ ์ฒ๋ฆฌ: ์๋ฌธ์ํ, ํน์ ๋ฌธ์ ์ ๊ฑฐ, ๋ถ์ฉ์ด ์ ๊ฑฐ
text = text.lower()
text = re.sub(r'[^\w\s]', '', text) #ํน์ ๋ฌธ์ ์ ๊ฑฐ
words = text.split() # ๊ณต๋ฐฑ์ผ๋ก ๋๋ ์ค
words = [word for word in words if word not in korean_stopwords and len(word) > 1] # ๋ถ์ฉ์ดX, ๊ธธ์ด๊ฐ 1๋ณด๋ค ํผ
return words
# ๋น๋ ๋ถ์ ๊ฒฐ๊ณผ ์ ์ฅ
word_frequencies = []
for file_path in file_paths:
with open(file_path, 'r', encoding='utf-8') as file:
text = file.read()
words = process_text(text)
word_freq = Counter(words) # ๋น๋ ๋ถ์
word_frequencies.append(word_freq) # ์ถ๊ฐํจ
# ํ์ผ๋ณ๋ก ๊ฐ์ฅ ์์ฃผ ๋ฑ์ฅํ ์์ 10๊ฐ ๋จ์ด ์ถ๋ ฅ
for i, freq in enumerate(word_frequencies):
print(f"\nํ์ผ {i+1}์ ์์ 10๊ฐ ๋จ์ด:")
print(freq.most_common(10))
# ์๊ฐํ: ํ์ผ๋ณ ์์ 10๊ฐ ๋จ์ด ๋น๋
for i, freq in enumerate(word_frequencies):
common_words = freq.most_common(10)
words, counts = zip(*common_words)
plt.figure(figsize=(10, 5))
plt.bar(words, counts)
plt.title(f'ํ์ผ {i+1} ์์ 10๊ฐ ๋จ์ด ๋น๋')
plt.xticks(rotation=45)
plt.show()
ํ์ผ 1์ ์์ 10๊ฐ ๋จ์ด:
[('์ ํ', 11), ('๊ธ๋ก๋ฒ', 10), ('์์ฅ', 7), ('๊ธฐ์ ', 5), ('๋ฐ๋์ฒด', 5), ('์ผ์ฑ์ ์', 4), ('์ค๋งํธํฐ', 4), ('๋์คํ๋ ์ด', 4), ('๊ฐ๋ ฅํ', 4), ('a์ฌ', 4)]
ํ์ผ 2์ ์์ 10๊ฐ ๋จ์ด:
[('์์ต๋๋ค', 19), ('๋ฐ๋์ฒด', 11), ('๊ธ๋ก๋ฒ', 11), ('์ฌ์
', 8), ('๋์คํ๋ ์ด', 7), ('์ค๋งํธํฐ', 7), ('์ผ์ฑ์ ์๋', 6), ('๋ค์ํ', 6), ('ํนํ', 6), ('๊ธฐ์ ', 5)]
ํ์ผ 3์ ์์ 10๊ฐ ๋จ์ด:
[('์์ต๋๋ค', 20), ('๊ธ๋ก๋ฒ', 12), ('์ผ์ฑ์ ์๋', 11), ('์์ผ๋ฉฐ', 11), ('๋ฐ๋์ฒด', 10), ('์ค๋งํธํฐ', 6), ('์์ฅ', 6), ('๊ธฐ์ ', 6), ('๋์คํ๋ ์ด', 5), ('๋ํ', 5)]
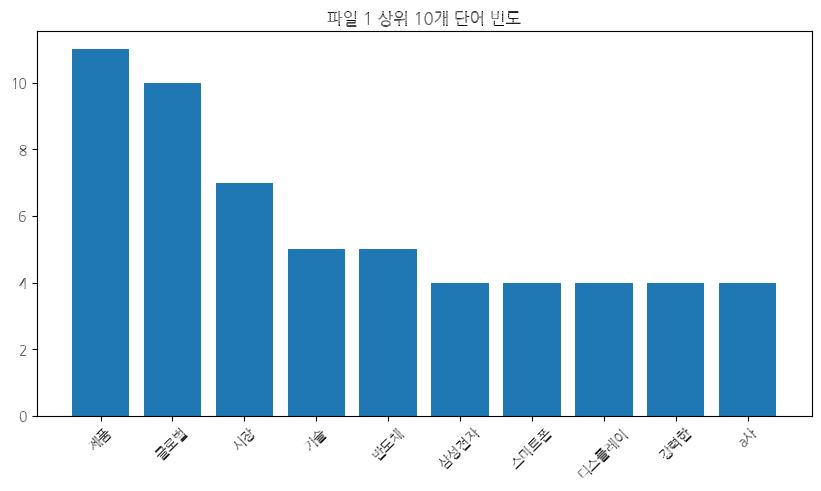
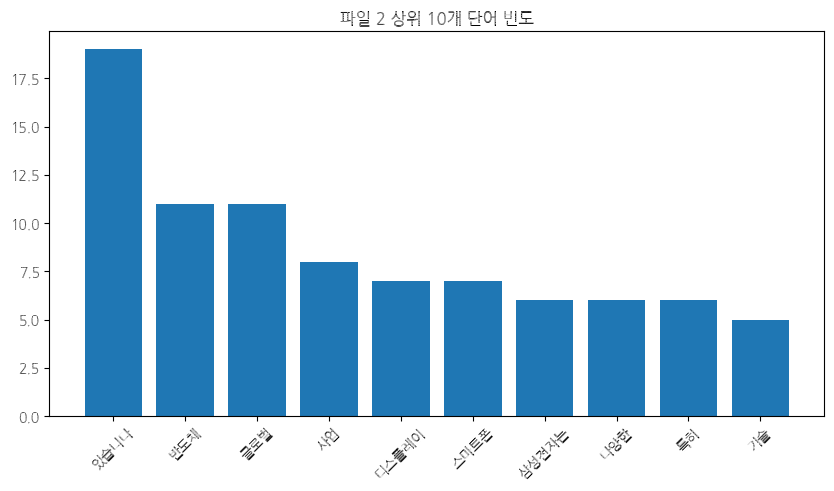
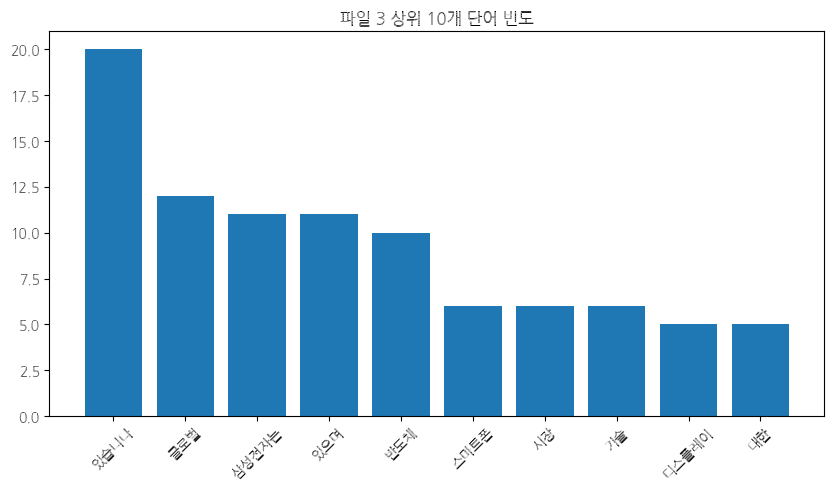
# ํ๊ตญ์ด ๋ถ์ฉ์ด ์ถ๊ฐ
# ์๋์ผ๋ก ์ ์ํ ํ๊ตญ์ด ๋ถ์ฉ์ด ๋ฆฌ์คํธ
korean_stopwords = { '์์ต๋๋ค', '์์ผ๋ฉฐ', '๋ํ',
'์', '๊ฐ', '์ด', '์', '๋ค', '๋', '์ข', '์', '๊ฑ', '๊ณผ', '๋', '๋ฅผ', '์ผ๋ก',
'์', '์', '์', 'ํ', 'ํ๋ค', '์์', '๊ฒ', '๋ฐ', '์ํด', '๊ทธ', '๋๋ค'
}
additional_stopwords = {'๊ฐ์ ', '์ฝ์ ', '๊ฒฝ์์ฌ'} # ๋ถ์์ ๋ถํ์ํ ๋จ์ด ์ถ๊ฐ
korean_stopwords.update(additional_stopwords)
# ํ์ผ๋ณ ํ
์คํธ ์ฒ๋ฆฌ ๋ฐ ๋จ์ด ๋น๋ ๊ณ์ฐ
def process_text(text):
# ํ
์คํธ ์ ์ฒ๋ฆฌ: ์๋ฌธ์ํ, ํน์ ๋ฌธ์ ์ ๊ฑฐ, ๋ถ์ฉ์ด ์ ๊ฑฐ
text = text.lower()
text = re.sub(r'[^\w\s]', '', text) #ํน์ ๋ฌธ์ ์ ๊ฑฐ
words = text.split() # ๊ณต๋ฐฑ์ผ๋ก ๋๋ ์ค
words = [word for word in words if word not in korean_stopwords and len(word) > 1] # ๋ถ์ฉ์ดX, ๊ธธ์ด๊ฐ 1๋ณด๋ค ํผ
return words
# ๋น๋ ๋ถ์ ๊ฒฐ๊ณผ ์ ์ฅ
word_frequencies = []
for file_path in file_paths:
with open(file_path, 'r', encoding='utf-8') as file:
text = file.read()
words = process_text(text)
word_freq = Counter(words) # ๋น๋ ๋ถ์
word_frequencies.append(word_freq) # ์ถ๊ฐํจ
# ํ์ผ๋ณ๋ก ๊ฐ์ฅ ์์ฃผ ๋ฑ์ฅํ ์์ 10๊ฐ ๋จ์ด ์ถ๋ ฅ
for i, freq in enumerate(word_frequencies):
print(f"\nํ์ผ {i+1}์ ์์ 10๊ฐ ๋จ์ด:")
print(freq.most_common(10))
# ์๊ฐํ: ํ์ผ๋ณ ์์ 10๊ฐ ๋จ์ด ๋น๋
for i, freq in enumerate(word_frequencies):
common_words = freq.most_common(10)
words, counts = zip(*common_words)
plt.figure(figsize=(10, 5))
plt.bar(words, counts)
plt.title(f'ํ์ผ {i+1} ์์ 10๊ฐ ๋จ์ด ๋น๋')
plt.xticks(rotation=45)
plt.show()
ํ์ผ 1์ ์์ 10๊ฐ ๋จ์ด:
[('์ ํ', 11), ('๊ธ๋ก๋ฒ', 10), ('์์ฅ', 7), ('๊ธฐ์ ', 5), ('๋ฐ๋์ฒด', 5), ('์ผ์ฑ์ ์', 4), ('์ค๋งํธํฐ', 4), ('๋์คํ๋ ์ด', 4), ('๊ฐ๋ ฅํ', 4), ('a์ฌ', 4)]
ํ์ผ 2์ ์์ 10๊ฐ ๋จ์ด:
[('๋ฐ๋์ฒด', 11), ('๊ธ๋ก๋ฒ', 11), ('์ฌ์
', 8), ('๋์คํ๋ ์ด', 7), ('์ค๋งํธํฐ', 7), ('์ผ์ฑ์ ์๋', 6), ('๋ค์ํ', 6), ('ํนํ', 6), ('๊ธฐ์ ', 5), ('๋คํธ์ํฌ', 5)]
ํ์ผ 3์ ์์ 10๊ฐ ๋จ์ด:
[('๊ธ๋ก๋ฒ', 12), ('์ผ์ฑ์ ์๋', 11), ('๋ฐ๋์ฒด', 10), ('์ค๋งํธํฐ', 6), ('์์ฅ', 6), ('๊ธฐ์ ', 6), ('๋์คํ๋ ์ด', 5), ('๋ชจ๋ฐ์ผ', 5), ('๋ค์ํ', 5), ('ํนํ', 5)]
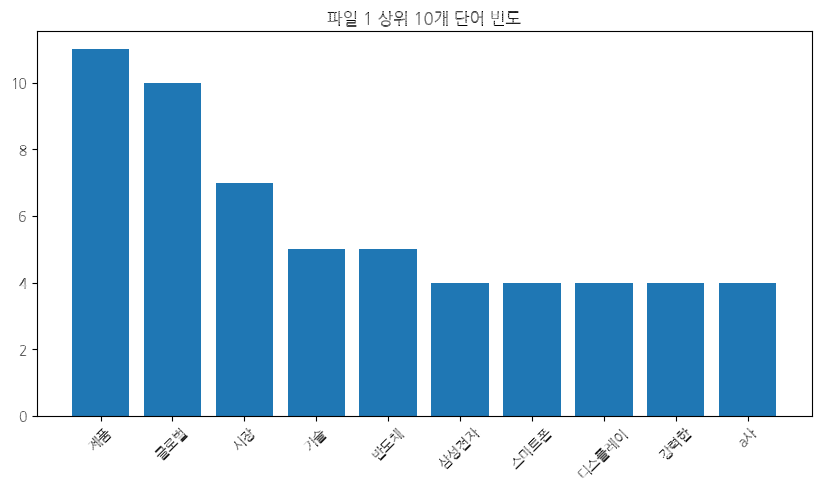

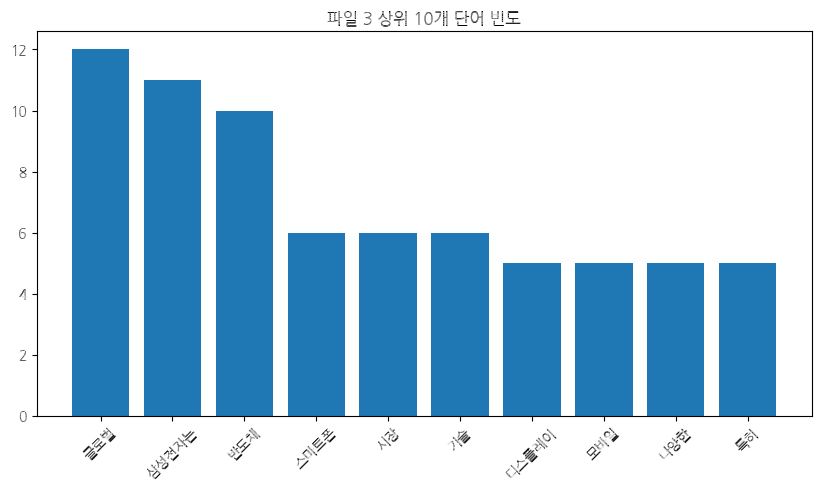
3-6 [๋ ๋ฒจ์ ์ค์ต 1] ๋ถ์ฉ์ด ํ์ผ์ ๋ง๋ค์ด์ ์ด๋ฅผ ๋ถ๋ฌ์์ ์ต์ข ์ ์ผ๋ก ๊ทธ๋ํ๋ฅผ ํ์ธํ๋ค.
```
์ฝ๋
```
๋ก ์ฌ๋ฆฌ๊ธฐ
names = ['Alice', 'Bob', 'Charlie']
ages = [25, 30, 35]
cities = ['New York', 'London', 'Paris']
for idx, i in enumerate(ages):
print(idx, i)
0 25
1 30
2 35
person_info = list(zip(names, ages, cities))
print(person_info)
[('Alice', 25, 'New York'), ('Bob', 30, 'London'), ('Charlie', 35, 'Paris')]
https://ldjwj.github.io/CLASS_PY_LIB_START/PYLIB_03_02_alice_extreme_V11_2411.html
unit01_02_alice_extreme_V11_2411
ldjwj.github.io
set ์งํฉ ์ค๋ณต ์ ๊ฑฐ๋จ
## ์งํฉ ํ์ธ
s2 = set([1,2,3,4,5,1,2])
s2
{1, 2, 3, 4, 5}
### ๋ถ์ฉ์ด ๋จ์ด ์ถ๊ฐ
x_words = set(STOPWORDS)
x_words.add("said")
x_words
Lexical dispersion is a measure of how frequently a word appears across the parts of a corpus.
Result)
https://openai.com/index/openai-api/
https://platform.openai.com/docs/overview
https://rowan-sail-868.notion.site/ChatGPT-API-1337d480b59380ec918bfe4d6c0f6c41
ChatGPT API ๊ธฐ๋ณธ ์์ํ๊ธฐ | Notion
1-1 ChatGPT API๋ ๋ฌด์์ธ๊ฐ์?
rowan-sail-868.notion.site
5๋ฌ๋ฌ ์ถฉ์
[์คํธ๋ฆผ๋ฆฟ] Github๋ก ์ฐ๋ํด์ ํ์ด์ง Deployํ๊ธฐ
Github์ ์ฐ๋ํด์ App ๋ฐฐํฌํ๊ธฐ.
velog.io